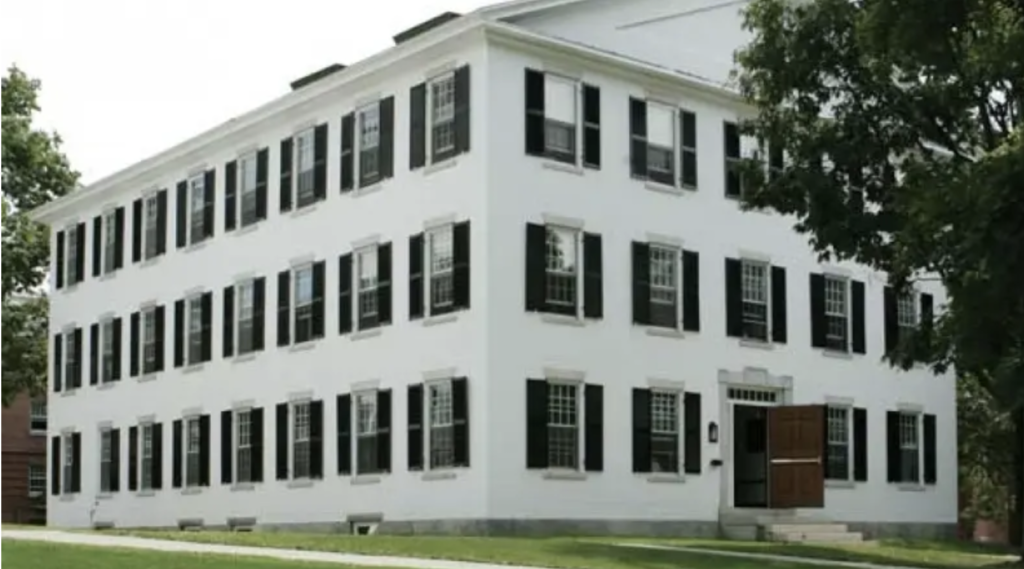
Dr. Ruth Chang gave a presentation to the wider Dartmouth community with facilitation from the Philosophy Department on April 4. Her presentation about Artificial Intelligence alignment, confused by her inadequate familiarity with machine learning, presents no actionable solution to the alignment problem and further obfuscates the problem.
AI alignment is the process of encoding human values and goals into an intelligent system. Given the (not really) imminent arrival of superhuman general artificial intelligence, some (misguided) alarmists believe it is essential to align AI with humans to prevent an extinction-level catastrophe. Chang motivates her solutions to the alignment problem with an already shaky assumption that alignment is a valid and important problem to solve.
Dr. Chang identifies two fundamental mistakes with the state of AI today:
1. We can use non-evaluative proxies for evaluative goals
2. Tradeoffs between different qualities have clear answers.
In non-engineer talk, problem 1 essentially states that we don’t train our models today with the data that we care about, but rather proxies for them. One useful example she uses is the failed Amazon resume screening disaster. When hiring managers started relying on AI to screen resumes, because the model was trained on previously hired individuals (who happened to be predominantly male), it learned to discriminate against women. The evaluative goal in this case was to hire those who were most qualified. Instead, because the AI was trained on non-evaluative proxies (such as gender), it gave us the wrong answers.
Her solution to this problem is technologically rather crude. Instead of feeding a resume into the model, we can pass in explicit value judgments previously made by humans. So the model would learn from an expert panel of humans who determine what makes a good candidate. The model would also directly predict the value judgment of humans (like loyalty and smartness for example) instead of a direct hire or no hire decision.
Not only is her solution reminiscent of McCarthy and Minsky’s failed MIT AI project, but it also makes little sense theoretically. According to her own Amazon example, the same resume is used to render a decision about a candidate by both an evaluative agent (human) and a non-evaluative agent (AI). Since the input data is the same, one might not find it controversial to say that the computations performed on the data are the problem, not necessarily the data itself.
While AI works by back-propagating on a series of artificial neurons, human brains operate with a collection of complex, interworking neural circuits. We should never expect an AI that makes decisions using very complex statistics to be aligned with a biological system that uses a fundamentally different mode of computation. When the input is held constant, we should not blame the fault of one system on the quality of the input data rather than the computation being performed on it.
When questioned about this simple objection, Dr. Chang displayed a surprising unfamiliarity with back-propagation, which has been the fundamental industry standard mode of training machine learning models for the past decade. AI isn’t making non-evaluative judgments because we’re training it with the wrong data (although that certainly happens), it’s making non-evaluative judgments because it’s making non-evaluative judgments! This would be the equivalent of transplanting human eyes on rhesus macaques and then wondering why they still can’t read all of a sudden: it’s a total non-starter. The problem lies with the brain itself, not the input.
Even theoretically, there’s no concrete reason to believe that a machine learning model trained on evaluative data would be aligned with human judgment. Reinforcement learning algorithms such as DecisionTransformers which directly learn from human judgments similar to how Dr. Chang’s solution might learn, still display incredibly inhuman strategies and behaviors in real-life deployments. The problem is only exacerbated when introducing self-play into the dynamic when considering DeepMind’s AlphaStar or MuZero.
Another antiquated example she lists delineates AI and humans in the amount of context we can use to make decisions. One of her examples held that a human could look at Dartmouth on a resume and pull in outside information like “Oh, he’s probably a smart student” while AI could not. Perhaps this was true for crude multi-layer perceptrons. However modern deep learning models could easily incorporate these contexts into their decision-making. I urge the reader to log into ChatGPT and ask “What might the fact that a person attended Dartmouth say about that person.”
When it comes down to it, all Machine Learning models now are a form of information compression: an input is given, which is then compressed to a lower-dimensional representation, which we then use to make decisions and predictions about the data. You would be hard-pressed to find any serious neuroscientist (serious meaning those who agree with me) who would agree that this is also how humans make value judgments. Dr. Chang’s suggestions are a band-aid solution.
However, given that we don’t yet understand how to build human-like AI, we might be tempted to listen to Dr. Chang’s prescriptions as a band-aid solution. But the bandaid is very porous. Even from a practical standpoint, her solutions are impractical to implement. We engineers use the data we use to train models because they are widely available (or otherwise cheap to collect) and easily transformable into numbers. It might seem easy to gather human-made value-based decision data when it comes to resumes but now try collecting the same kind of alignment data on the order of The Pile, 825GBs of text data collected from books and the internet. The task becomes borderline impossible. Even if the dataset is small, certain domains of decisions make this kind of training impossible to do. How could you gather directly value-based decision data when it comes to fundamental physics or medicine discovery (both of which also require alignment to reduce the risk of bad-actor use)? What about meta-cognitive models like language models that don’t make decisions at all, but rather try and predict the next best token? It is hard to imagine how her model can be extended in such cases.
A more fundamental issue present in the lack of clarity in her analysis is the decapitation of the aim of philosophy. From the very beginning, philosophy was never meant to be a dedicated field. Ancient philosophers were also mathematicians, astronomers, literary artists, and astrologers in addition to being philosophers. Philosophy helped technologists think fundamentally about their technology, about its impact on the world, and what it implied for our existence. When philosophy started parting ways with technology which grounded it in reality, we started getting phenomenology, existentialism, nihilism, and postmodern philosophies which have only yielded thoughts no reasonably functioning human being should embody.
When philosophy became professionalized at the leash of academia and the federal grant system, it became increasingly cheated: infested with incremental research that does not contribute to humanity and filled with semantic debates, manufactured definitions, and weak claims. Although making zero contributions to society is certainly better than making negative contributions, it is a sad state for a field that used to be filled with based philosophers.
Dr. Chang is incredibly accomplished in law and the philosophy of values. Her CV speaks for itself. I’m certain that she is a good-faith academic, demonstrating attempts to understand computer scientists’ perspectives and incorporating them into her theories about the future of AI. The problem is not necessarily with her theories but rather with the stunted bureaucracy (in the form of AI ethics boards and activists) that poorly understands both her theories and the technology with a strangling grasp on the work of technologists. These ethics boards, who I call the “axis of uneducated and incapable,” should not be allowed to slow down the work of men and women who are capable of bringing about change. For not all change is good, but any change is better than stagnation.
Be the first to comment on "The Death of Philosophy at the Hands of Artificial Intelligence"